Big Data in Transportation
Overview[edit | edit source]
Big data is an approach and attitude to analyzing data, with an acknowledgement that combining data from multiple sources could lead to better decisions.[1] As emerging transportation technologies grow in popularity and abundance, transportation data is becoming more precise and available than it has ever been. Organizing and processing data at this growing scale in a consistent and meaningful manner is challenging for public agencies. Improving database capacity and updating procurement policies will help agencies maximize the potential of big data. Agencies who have succeeded at transitioning data platforms include the Kentucky Transportation Cabinet, the City of Portland, and the City of Columbus. Key “players in the field” include private transportation companies and software companies focusing on data storage and processing.
Analysis of Implications[edit | edit source]
Policy and Planning[edit | edit source]
Big data can support equity in transportation planning and engineering. Cell phone location data is generally representative across income and racial groups. Big data can help verify assumptions that planners and engineers make about the community needs and demographics. Big data can help transportation professionals understand the broader connection to individual corridors and intersections. Systemic approaches can be a more cost-effective, efficient, and consistent way to meeting transportation needs. It allows transportation professionals to quantify benefits to users, rather than motor vehicles or buses. Cell phone data collected by tech companies can be used by transportation professionals to analyze travel patterns and plan for new infrastructure.
Technology[edit | edit source]
Transportation data is better than it has ever been, and public agencies have an opportunity to institute the data-related reforms that will help them deliver more equitable, sustainable, and efficient projects. However, challenges in harnessing this data efficiently include the following:
- A lack of governmental capacity and standards
- Outdated procurement procedures
- Risk of privacy breaches
Governments will need to improve public sector capacity, update procurement policies, and consider new systems to centrally manage sensitive private data.[2] Current databases will not be able to store the new data that is becoming available because the data is too varied, large, updates irregularly and/or often, and the formatting can change frequently.[1]
Big data can impact transportation in the following areas:
- Asset management: creating a more efficient management of assets and a more reliable network
- Traffic management: aiding prediction and management of congestion
- Logistics and supply chain management: providing route optimization and forecast modification
- Public transportation improvement: revealing public transit gaps and expansion opportunities
- Roadway infrastructure: Allowing development of apps to enable residents to identify needs in roadway infrastructure and conducting before-and-after studies to weigh benefits of projects such as road diets
- Roadway maintenance: Identifying needs and directing maintenance resources to most critical needs[3][4]
Key Issues[edit | edit source]
Successful, safe, and equitable application of big data will require resolution of the following concerns:
- Data Security: Although most data owned by public agencies is public information, ransomware attacks are a very real threat to local governments.
- Data Privacy: This is a growing concern as smartphones and social media continuously track users' location and other data. Location data is extremely precise and can be used to identify a person based on their home and work locations, or other frequently traveled destinations.[5]
- Data Standardization: Some areas of the industry, such as transit and bikeshare, are far along in terms of standard data. The General Transit Feed Specification (GTFS) and the General Bikeshare Feed Specification (GBFS) allow cities to share standardized bikeshare and transit data with other agencies and consultants. OpenStreetMap, which is used as a linear reference system, is starting to become a standard for infrastructure data as well. However, a lot of transportation data (e.g. vehicle counts) is still supplier-specific.
- Limitations of Data: Big data is not perfectly accurate and tends to work in certain conditions better than others. For example, StreetLight data tends to overestimate vehicle volumes in high pedestrian and high transit areas.[6] Understanding the metadata is essential to make sure that analysis can yield useful results.
- Biases: Data and datasets are not perfectly objective. For example, Strava data is biased towards young, wealthy, active individuals. In addition, a lot of data is collected through social media, which creates a bias towards people who are avid social media users (e.g. young, male, tech-savvy) and skews towards activities that are socially desirable (i.e. "participants may only log bicycle trips that are sufficiently fast or long to record and share as an accomplishment").[7]
Case Studies[edit | edit source]
LYNX: SR 436 Transit Corridor Study[edit | edit source]
LYNX, the transit agency serving the Orlando, Florida, metropolitan region considered a range of alternatives to increase the frequency and quality of transit service. Through the use of open-source data formats and software, the LYNX team (including Kittelson & Associates, Inc. and Omnimodal, LLC) was able to simulate the impact of its proposed alternatives on a trip-by-trip basis. They built a trip routing engine using OpenTripPlanner, an open source trip planning software. OpenTripPlanner requires an OpenStreetMap file and a GTFS transit schedule dataset. Next, two trip routing “passes” were performed for all trips interacting with SR 436. The first pass used a baseline (existing) GTFS file. The second pass used a GTFS file that reflected the addition of the proposed alternative. The detailed trip routing outputs were used to measure the impact of the proposed route on riders’ experiences. The tools and data sources used for this study could be applied to larger geographies and help inform system redesign studies.[8]
Kentucky Transportation Cabinet[edit | edit source]
The Kentucky Transportation Cabinet (KYTC) wanted to leverage real-time data from snowplows outfitted with automatic vehicle location (AVL) technology to control snow and ice costs and improve roadway safety. KYTC added Waze and doppler data and their existing system could not handle it. KYTC ended up partnering with Apache Spark for processing data and Hadoop for warehousing to create a new data database and processing architecture. The current system cut the records processing time from 45 minutes to 35 seconds. This data helps route maintenance trucks to make the most efficient use of tax dollars [9]
Portland Urban Data Lake[edit | edit source]
The City of Portland created the Portland Urban Data Lake (PUDL) pilot which collects and stores data from a variety of sources; develops analytics to create new insights from the data; and explores technologies and architectures for providing standardized, documented access to data for public sector agencies and local innovators.[10] PUDL handles various data including data from IoT devices, origin-destination data, Waze traffic data, pedestrian counts and more.[1]
Smart Columbus[edit | edit source]
The City of Columbus, Ohio won the U.S. Department of Transportation’s first-ever Smart City Challenge. Columbus was awarded $50 million in grant funding to develop the “Smart Columbus” project. This project goes beyond the typical open data policies by developing and hosting two major data sharing projects: the data and analytics hub, “Smart Columbus OS,” and the application-focused, “Integrated Data Exchange,” or IDE. The Smart Columbus OS system hosts over 3,000 publicly available datasets including traffic characteristics, infrastructure inventory, parking locations, and emergency response times. The data has been used to show riders where their bus is in real time, improve transportation access for older populations, understand parking demand, and discover safety solutions.[11] The IDE supports IoT devises and business applications by providing a unified data platform that can integrate with emerging technologies and business that use them. The goal is to gather data from multiple IoT sources, ensure the privacy of the data, and govern access to the data to ensure usability. Both platforms promote open data sharing among business partners, researchers, and everyday users.[1]
Twelve Million Phones, One Dataset, Zero Privacy[edit | edit source]
The Times Privacy Project investigated location data privacy concerns. They found that in most cases, a person could be identified by their home and office locations using the data, and that "describing location data as anonymous is "a completely false claim" because "really precise, longitudinal geographical information is absolutely impossible to anonymize." Additionally, "if a private company is legally collecting location data, they're free to spread it or share it however they want." Creating federal and state privacy laws may help protect this data.[12]
Players in the Field[edit | edit source]
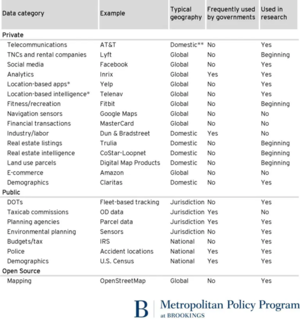
- State and local agencies are using this data to make planning decisions. Examples are provided in the cases studies.
- Sidewalk Labs is an urban innovation company that uses big data to "reimagine cities to improve quality of life."[14]
- There is a wide array of transportation data currently collected and available. The image on the right shows some emerging datasets. This includes data obtained from third parties (e.g. Lyft, INRIX, Amazon), and data collected by public agencies (e.g. signal traffic counts, crash data).[15]
- Other players include software companies that are involved in partnering with public agencies to process or store transportation data. These include the following:
- Apache Hadoop - open-source software for reliable, scalable, distributed computing
- Apache Spark - unified analytics engine for large-scale data processing
- Quantzig - end-to-end data modeling
- Microsoft - breaking new ground in ubiquitous computing and advanced data analytics. Partnered with the City of Seattle to develop an architecture called the Trusted Data Platform to enable cross-functional sharing of data and assets between public and private entities as part of the Smart Seattle initiative.[16]
- ↑ 1.0 1.1 1.2 1.3 National Academies of Sciences, Engineering, and Medicine 2020. “Guidebook for Managing Data from Emerging Technologies for Transportation”. Washington, DC: The National Academies Press. Accessed on September 4, 2020. https://doi.org/10.17226/25844.
- ↑ Tomer, Adie and Shivaram, Ranjitha (July 20, 2017). “Modernizing government’s approach to transportation and land use data: Challenges and opportunities.” Accessed On September 4, 2020. https://www.brookings.edu/research/modernizing-approach-to-data/
- ↑ Clifford, Tori. “5 Urban Transportation Challenges that Big Data Can Help You Solve.” Accessed on September 14, 2020. https://www.streetlightdata.com/5-urban-transportation-challenges-that-big-data-can-help-you-solve/#:~:text=Big%20Data%20can%20help%20reveal,infrastructure%20improvements%20on%20vehicle%20traffic.&text=As%20with%20other%20types%20of,to%20locate%20bike%20share%20stations
- ↑ Joshi, Naveen (September 19, 2017). “This is why big data in transportation is a big deal.” Accessed on September 4, 2020. https://www.allerin.com/blog/this-is-why-big-data-in-transportation-is-a-big-deal
- ↑ https://www.nytimes.com/interactive/2019/12/19/opinion/location-tracking-cell-phone.html
- ↑ https://www.fehrandpeers.com/transformative-data-collection-solution/
- ↑ https://safed.vtti.vt.edu/wp-content/uploads/2020/07/02-026_Final-Research-Report_Final.pdf
- ↑ https://www.lynxsr436.com/the-story-of-a-trip/
- ↑ Kanowitz, Stephanie (November 29, 2016). “Kentucky plows through big data on winter roadways.” Accessed on September 4, 2020. https://gcn.com/articles/2016/11/29/kentucky-intelligent-transportation.aspx
- ↑ Hill, Anne and Kerr, Michael. “Portland Urban Data Lake (PUDL). Accessed on September 4, 2020 https://www.portlandoregon.gov/transportation/article/681572
- ↑ https://www.smartcolumbusos.com/about/about-smart-columbus
- ↑ https://www.nytimes.com/interactive/2019/12/19/opinion/location-tracking-cell-phone.html
- ↑ Tomer, Adie and Shivaram, Ranjitha (July 20, 2017). “Modernizing government’s approach to transportation and land use data: Challenges and opportunities.” Accessed On September 4, 2020. https://www.brookings.edu/research/modernizing-approach-to-data/
- ↑ https://www.sidewalklabs.com/
- ↑ Tomer, Adie and Shivaram, Ranjitha (July 20, 2017). “Modernizing government’s approach to transportation and land use data: Challenges and opportunities.” Accessed On September 4, 2020. https://www.brookings.edu/research/modernizing-approach-to-data/
- ↑ https://www.transportation.gov/sites/dot.gov/files/docs/WA%20Seattle.pdf